The focus of research is moving from treating illnesses to avoiding them completely in a society where healthcare expenses are steadily soaring and millions of people are living with chronic conditions. Artificial Intelligence (AI) stands at the forefront of this revolution due to its unprecedented capabilities to identify health risks before they develop into serious conditions.
However, how exactly is AI changing healthcare prevention? AI is changing the way we approach disease prevention and health maintenance, from advanced screening tools to well-designed immunization programs and ongoing health monitoring.
Therefore, in this piece of content, I will explain uses of AI in preventive healthcare for screening, vaccination and detection before it becomes chronic.
The Evolution of AI in Healthcare
Do you remember when healthcare was primarily reactive? You’d get sick, visit a doctor, and receive treatment. That model is rapidly becoming outdated, largely to AI.
The AI in healthcare is more and more proactive, seeing possible problems before symptoms show up and taking early action to stop the progression of diseases.
1. From Reactive to Preventive Healthcare Models
The shift from reactive to preventive care represents one of the most significant paradigm shifts in modern medicine. Traditional healthcare systems typically engage with patients only after symptoms appear, often when diseases have already progressed. This approach not only impacts patient outcomes but also strains healthcare resources.
This model has been fundamentally rethought as because of AI. Artificial intelligence (AI) can find subtle patterns and risk factors that human clinicians might overlook by analyzing enormous amounts of health data, such as genetic information, lifestyle factors, and electronic health records. This makes it possible to implement focused treatments before illnesses show clinical symptoms.
Dr. Eric Topol, a leading AI-in-medicine researcher, notes:
“AI’s ability to synthesize data at scale is transforming medicine from a reactive art to a proactive science.“
2. Key Technologies Driving AI-Powered Prevention
Technology | Role in Prevention | Example |
---|---|---|
Machine Learning | Improves risk prediction accuracy through continuous learning. | Predicting diabetes risk via lifestyle data. |
Deep Learning | Detects complex patterns in medical images and genomics. | Identifying tumors in MRI scans. |
Natural Language Processing (NLP) | Extracts insights from unstructured clinical notes. | Flagging drug interactions in patient histories. |
Several core technologies form the backbone of AI’s preventive capabilities:
Machine learning algorithms can improve their accuracy over time by using new data, which results in risk assessments that are more accurate.
Deep learning is a branch of machine learning which is particularly good at finding intricate patterns in high-dimensional data, such as genetic sequences and medical images.
Natural language processing makes previously unreachable data available for preventive analysis by assisting to extract insightful information from unstructured medical records and research literature.
Instead of functioning independently, these technologies combine to form comprehensive systems that are capable of concurrently analyzing health from a variety of perspectives.
Screening Technologies of AI in Preventive Healthcare
Screening serves as the first line of defense in preventive healthcare, and AI is enhancing its effectiveness and accessibility. Traditional screening programs often struggled with issues of sensitivity, specificity, and scalability. AI addresses these challenges head-on.
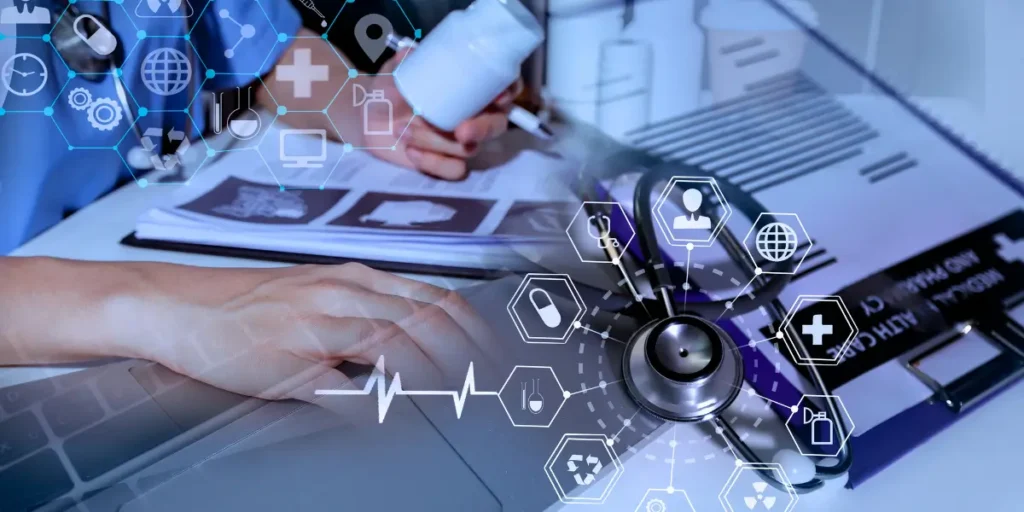
1. Medical Imaging Analysis and Early Detection
A radiologist likely spent minutes reviewing an X-ray, mammography, or CT scan. Machines specifically know as Internet of things (IoT) can examine photos in seconds and find minor problems that humans cannot.
In mammography, AI algorithms can identify suspicious areas that can indicate early-stage breast cancer, flagging them for radiologist review. Studies show that AI-assisted mammography screening can increase cancer detection rates by up to 13% while reducing false positives.
Similarly, a major cause of blindness, diabetic retinopathy, can be identified early with AI tools that analyze retinal scans, which lets swift action.
The technology broadens our understanding of early warning signs by identifying new patterns that correlate with disease risk rather than merely searching for well-known disease markers.
2. Genetic Screening and Personalized Risk Assessment
AI is getting better at interpreting the intricate code that our genes contain, which contains important hints about our health tendencies. AI can produce individualized risk profiles for a wide range of illnesses, from heart disease to different types of cancer, by evaluating genetic data.
These analyses go far beyond simple genetic determinism. Modern AI systems integrate genetic information with environmental factors, lifestyle choices, and social determinants of health to create holistic risk assessments.
For example, an algorithm may determine that you have a higher risk of type 2 diabetes due to your genetic profile, sedentary lifestyle, and eating habits. This would enable you to implement specific preventative measures.
Case Study: AI-Powered Cancer Screening
One of the most important parts of preventative healthcare is cancer screening, so let’s see how AI is changing that.
- Lung Cancer: AI-assisted low-dose CT scans detect early-stage tumors with remarkable accuracy. A study found that AI identified lung cancers a year before conventional method.
- Colorectal Cancer: AI tools analyze colonoscopy videos in real-time, improving polyp detection rates by up to 20%.
- Breast Cancer: AI in screening mammography has demonstrated increased cancer detection rates and fewer false positives.
Vaccination Programs of AI in Preventive Healthcare
Vaccines represent one of humanity’s greatest public health achievements. The advancements in AI is serving to maximize their impact through smarter deployment and development.
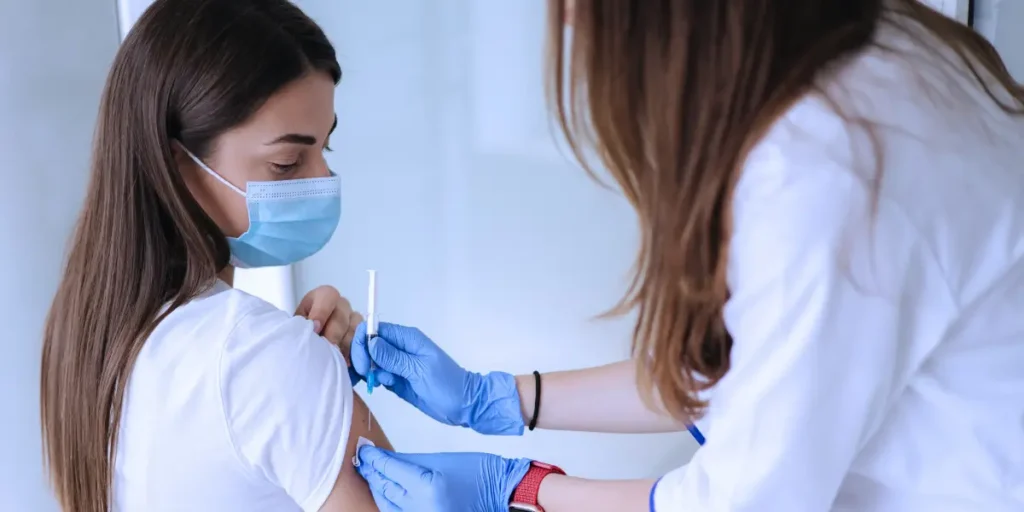
1. Predicting Outbreak Patterns and Vaccine Needs
Have you ever wondered how public health officials decide where to prioritize vaccine distribution? Increasingly, the answer involves AI. AI can predict disease outbreaks before they spread by examining a variety of data streams, like environmental factors, population mobility patterns, and social media mentions of symptoms.
Many AI models managed to accurately forecast regional outbreak patterns during the COVID-19 pandemic, which assisted authorities in allocating scarce vaccine supplies to the most vulnerable areas. As new variables are added and their predictive abilities are improved, these systems keep developing.
Beyond outbreak forecasting, AI aids in vaccine supply chain optimization by predicting demand trends and spotting possible distribution bottlenecks before they materialize.
This guarantees that the appropriate vaccinations arrive at the appropriate times and locations, addressing a logistical issue that has traditionally reduced the efficacy of vaccination programs.
2. Personalized Vaccination Schedules
Not everyone responds to vaccines in the same way, and increasingly, vaccination is moving away from one-size-fits-all approaches. AI helps create personalized vaccination schedules based on individual risk factors, immune status, and exposure likelihood.
For example, algorithms can identify high-risk individuals who can benefit from accelerated vaccination schedules or higher-dose formulations. These also made easier to determine optimal timing for booster shots based on waning immunity patterns specific to individual profiles.
3. The Role of AI in Vaccine Development
The development of new vaccines traditionally takes years, if not decades. AI is dramatically accelerating this timeline by streamlining multiple aspects of the development process by,
- Identifying promising antigenic targets.
- Optimizing clinical trial participant selection.
- Monitoring real-world vaccine performance to detect rare side effects.
During initial research phases, AI algorithms can identify promising antigenic targets by analyzing pathogen structures and predicting immune responses. In clinical trials, machine learning helps select optimal participant cohorts and predict potential adverse effects before they occur.
Most remarkably, AI can track the effectiveness of vaccines in the real world after they are deployed, detecting uncommon side effects and differences in efficacy between populations.
As a result, there is a feedback loop that influences both present vaccination use and upcoming research initiatives.
Early Detection Systems With AI in Preventive Healthcare
Beyond screening and vaccination, AI enables continuous health monitoring that can detect subtle changes indicating potential health issues.
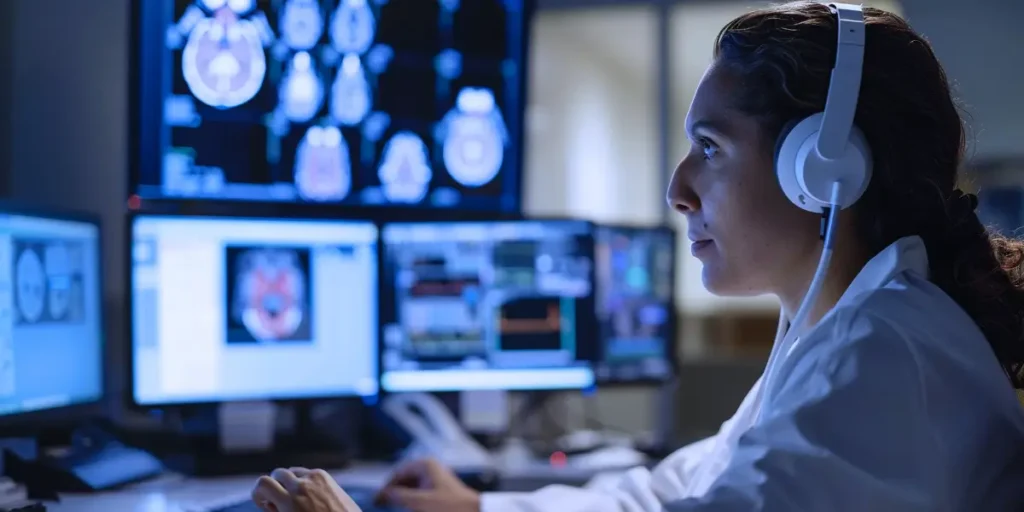
1. Wearable Technologies and Health Monitoring
The smartwatch on your wrist isn’t just counting steps – it can be serving as an AI-powered health sentinel. Modern wearable devices collect continuous data on various physiological parameters, from heart rate and sleep patterns to blood oxygen levels and even electrocardiogram readings.
Real-time analysis of these data streams by AI algorithms creates customized baselines and notifies users of significant variations. For example, algorithms can identify abnormal heartbeats that can be a sign of atrial fibrillation, a serious risk factor for stroke that frequently goes undetected.
Some systems use wearable data, environmental data, and self-reported symptoms to detect infectious diseases and mental health issues early.
A number of wearable technologies detected COVID-19 infections days before symptoms showed, suggesting early isolation and treatment.
2. Predictive Analytics for Chronic Disease Management
For those already managing chronic conditions, AI offers powerful tools to prevent complications and disease progression. Predictive analytics can forecast disease trajectories and identify early warning signs of deterioration, enabling timely interventions.
Predictive analytics improve chronic disease management:
- Diabetes: AI predicts hypoglycemic events hours in advance, enabling timely intervention.
- Heart Failure: AI forecasts decompensation events, potentially preventing hospitalizations.
Implementation Challenges and Ethical Considerations
Despite its enormous potential, implementing AI in preventive healthcare presents significant challenges that must be thoughtfully addressed.
1. Data Privacy and Security Concerns
Access to private health data is necessary for effective preventive AI, which brings up significant privacy issues. How can the advantages of thorough health monitoring be balanced with people’s right to privacy?
Strong consent procedures, open policies, and secure data handling are crucial cornerstones. New methods for deploying AI that protects privacy include federated learning, in which algorithms learn from data without the data ever leaving local systems.
Cybersecurity is equally important. Healthcare systems are more susceptible to algorithmic tampering and data breaches as a result of their growing reliance on AI for preventive care.
2. Ensuring Equitable Access to AI-Powered Healthcare
Making sure AI-powered prevention benefits everyone, not just privileged groups, is arguably the biggest challenge. Due to biases in the training data, many AI systems currently perform less accurately for underrepresented groups.
Access to AI-enhanced preventive care is also frequently influenced by variables such as insurance coverage, digital literacy, and geographic location.
Developing training datasets, designing deployment strategies that prioritize underserved communities, and creating culturally appropriate interfaces are all necessary steps in the intentional effort to address these disparities. AI can actually exacerbate current health disparities rather than lessen them in the absence of such measures.
Final Words
AI in preventive healthcare is opening up new avenues for earlier, more precise, and more thorough identification and management of health risks. These technologies provide effective tools in the fight to prevent disease rather than just treatment from advanced screening tools to well-designed immunization programs and ongoing monitoring systems.
However, careful execution is needed to realize this potential. We need to create suitable laws and regulations, guarantee fair access, and address data privacy issues. Above all, it’s necessary to keep in mind that AI tools are designed to complement human interaction, not to replace it.